Robopet fans’ dream comes true: a robot dog learned to walk in a single hour
The Dreamer programme created a new system that allows robots to learn by their prior experiences
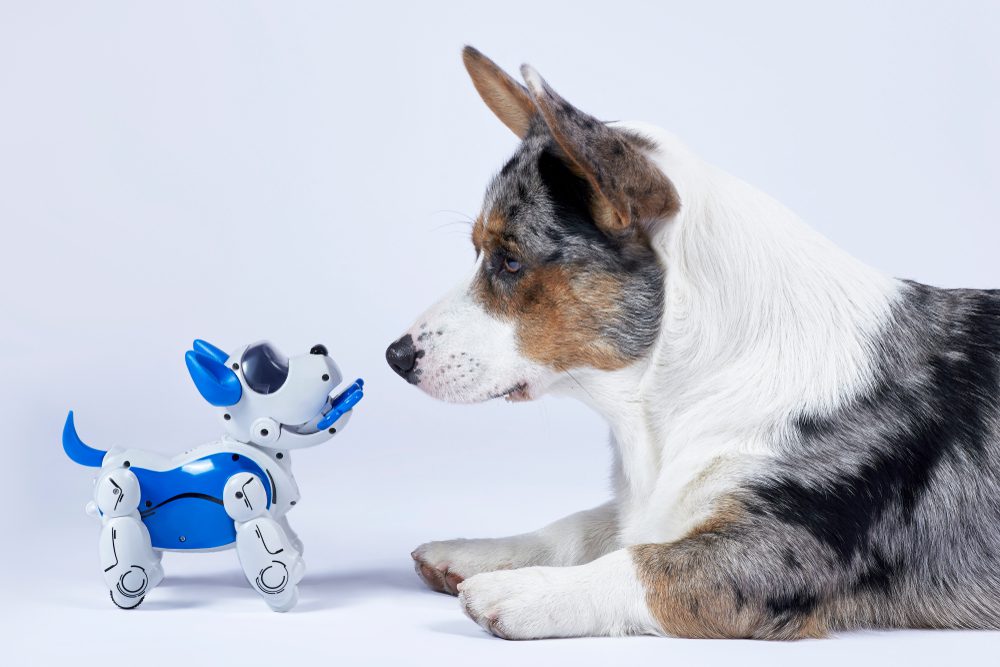
To educate robots without simulations or demonstrations, a research team from the University of California, Berkeley created a new algorithm based on reinforcement learning (RL).
The technique provides robots with a trial-and-error learning methodology that might be revolutionary for effective training of robots in the actual world. Reinforcement learning is used by the Dreamer programme to ‘train’ robots by providing ongoing feedback and rewarding them when a task is accomplished successfully.
To put the RL capabilities to the test, Dreamer was applied to four robots. In one hour a quadruped robot learned to walk, stand on its back, withstand pushes and roll over.
Additionally, using camera images, the scientists trained two robotic arms to pick and position objects, exceeding model-free units in their performance. When installed on a wheeled robot, Dreamer assisted it in using solely camera images to guide itself to a destination.
Reinforcement learning training was typically seen as ineffective, however the Dreamer method employs a small amount of interaction to generate the trial and error actions a robot takes to plan its movements, which is slightly different from standard deep reinforcement learning techniques.
Initial results are encouraging, but more research is required to determine how the algorithm will behave in various scenarios. Additionally, there are still issues with the amount of time needed to code each robot.